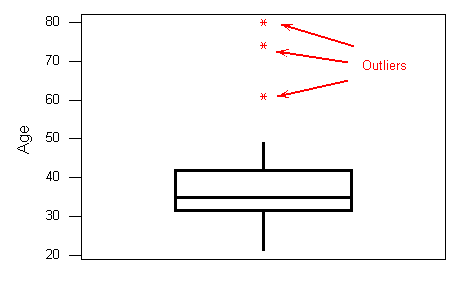
User-defined missing values on the original variable become system-missing values on the new variables. The result of the list follows, notice that SPSS marks user-missing values with a -9 in the listing. There is a -9 everywhere in the listing that there was a -9 in the data, so the value of the user-defined missing is preserved for the original variables (). values are numeric values that need to be defined as missing for SPSS. You might notice that some of the reaction times are -9 in the data below.
It is justifiable to exclude ‘outlier’ data points from statistical analysis for significance level of 0.005 or less according R.A. However, choosing a value of significance level for outlier detection is one of the problems. The second problem is that well-known statistical methods are used to detect outliers in a data set under the assumption that the data is generated by the Gaussian distribution. The second problem can be solved by applying the normalizing transformations. In the case of normally distributed data, the three sigma rule means that roughly 1 in 22 observations will differ by twice the standard deviation or more from the mean, and 1 in 370 will deviate by three times the standard deviation.
Standard Deviation Method
What does it mean to be an outlier?
An outlier is an observation that lies an abnormal distance from other values in a random sample from a population. In a sense, this definition leaves it up to the analyst (or a consensus process) to decide what will be considered abnormal. These points are often referred to as outliers.
Perhaps one outlier is enough to create a biased (statistical) pattern when the value is really extreme. Extreme values can be found out just by looking at the values in a data set, also based on past experience with a model species/system.
In most larger samplings of data, some data points will be further away from the sample mean than what is deemed reasonable. This can be due to incidental systematic error or flaws in the theory that generated an assumed family of probability distributions, or it may be that some observations are far from the center of the data. Outlier points can therefore indicate faulty data, erroneous procedures, or areas where a certain theory might not be valid. However, in large samples, a small number of outliers is to be expected (and not due to any anomalous condition).
British Dictionary definitions for outlier
When the possible range of data is known such as in age of humans measured in years. A human height value of 4.2 meters most be due to error and definitely will be discarded.
In this case, the median better reflects the temperature of a randomly sampled object (but not the temperature in the room) than the mean; naively interpreting the mean as “a typical sample”, equivalent to the median, is incorrect. As illustrated in this case, outliers may indicate data points that belong to a different population than the rest of the sample set. If the data set contains an odd number of points, this is easy to find – the median is the point which has the same number of points above as below it. However, if there are an even number of points, then, since there is no single middle point, the 2 middle points should be averaged to find the median.
How to Calculate Outliers
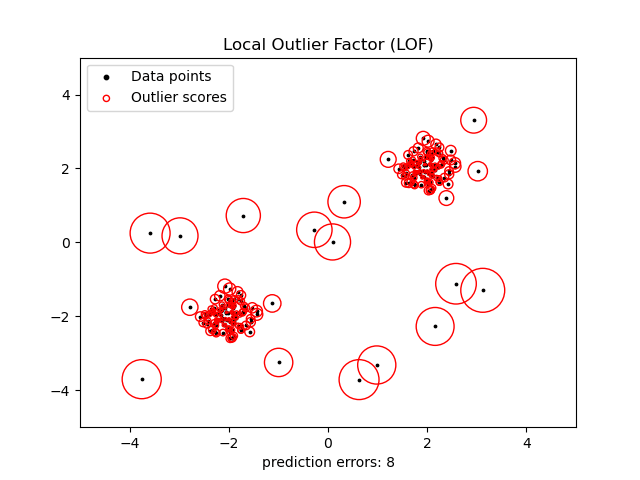
You will also notice that each row is labeled with a number (“1,” “2,” and so on). When you enter values for your data in the spreadsheet cells, each value will correspond to a specific variable (column) and a specific case (row). The list below illustrates how missing values are handled in assignment statements. The variable avgis based on the variables trial1 trial2 and trial3, and the variable avgris based on the variables trialr1 trialr2 and trialr3.
Also note that in general the validity of the results of a statistical analysis are always dependent on whether various statistical assumptions are satisfied. For some problems it is possible to design the experiment to ensure that the assumptions are satisfied but for other data sets it is not. Outlier values can come from poor recording of data during data collection in the field or during data coding and input in the office among others. There are cases where it is difficult to identify outlies, and there are case where they can be easily identified.
In short, descriptives used all of the valid data and performed the computations on all of the available data. This was also true for the next three variables containing user-missing values. Nevertheless, outliers can occur in a given dataset when the response is more than what is considered within the ‘norm’ in a population/sample. This normally happens when the response is more than 3 standard deviation away from the mean. Some statistical computations are moderately insensitive to “outliers” whereas others are very sensitive.
Instead, you should create a variable in your dataset that is used to identify each case—for example, a variable called StudentID. Values for each existing variable in your dataset will be missing (indicated by either a “.” or a blank cell) for your newly created case since you have not yet entered this information. Sometimes you may need to add new cases or delete existing cases from your dataset. For example, perhaps you notice that one observation in your data was accidentally left out of the dataset. In that situation, you would refer to the original data collection materials and enter the missing case into the dataset (as well as the associated values for each variable in the dataset).
- Data analyzers inspecting tables or figures may decide to exclude from data sets unusual data points named ‘outliers’.
- However, underlying mechanisms creating outlier data points are usually unknown.
- The outcome of statistical analyses will probably differ in analyses with versus without outlier data points.
What is another word for outlier?
Words related to outlier aberration, deviation, oddity, eccentricity, exception, quirk, anomaly, deviance, irregularity, outsider, nonconformist, maverick, original, eccentric, bohemian, dissident, dissenter, iconoclast, heretic.
Data analyzers inspecting tables or figures may decide to exclude from data sets unusual data points named ‘outliers’. The outcome of statistical analyses will probably differ in analyses with versus without outlier data points.
An outlier is an observation in a data set that lies a substantial distance from other observations. These unusual observations can have a disproportionate effect on statistical analysis, such as the mean, which can lead to misleading results. Outliers can provide useful information about your data or process, so it’s important to investigate them.
In this case it is statistically justifiable not to include such data points in the statistical analysis. The researcher must know what sounds reasonable and justifiable data for his analysis. Plotting a Boxplot diagram helps to identify where the outliers are coming from. The possibility should be considered that the underlying distribution of the data is not approximately normal, having “fat tails”. Even a slight difference in the fatness of the tails can make a large difference in the expected number of extreme values.
outlier
If the sample size is only 100, however, just three such outliers are already reason for concern, being more than 11 times the expected number. Naive interpretation of statistics derived from data sets that include outliers may be misleading.
You may use any value you choose to stand for a missing value, but be careful that you don’t choose a value for missing that already exists for the variable in the data set. For that reason many people choose negative numbers or large numbers to represent missing values. You may read raw data with user-missing values either as fixed field input or as free field input. When defined as such on a missing values command these values of -9 are treated asuser-missing values.
When you enter data into SPSS, you will need to make sure that you are entering values for each variable that correspond to the correct person or object in your sample. However, you should never rely on these pre-numbered rows for keeping track of the specific respondents in your sample. This is because the numbers for each row are visual guides only—they are not attached to specific lines of data, and thus cannot be used to identify specific cases in your data. Again, the row numbers in SPSS are not attached to specific lines of data and should not be used to identify certain cases.
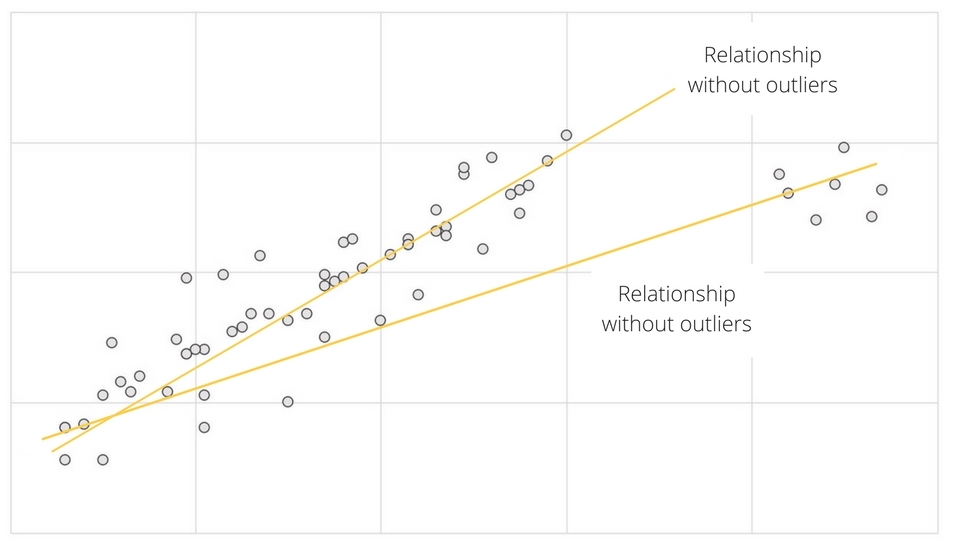
However, if the two middle points are the same number, the average, obviously, will be this number as well, which is also OK. As you see in the output below, descriptives computed the means using four observations for trial1 and trial2and six observations for trial3.
We would expect that it would do the computations based on the available data, and omit the missing values for each pair of variables. The crosstabs command only includes valid (non-missing data) in its tables. Cases containing a missing value for even one of the variables are not included in the table. Note that the percentages are computed based on just the non-missing cases. The compute command is used to create the new variables trialr1 through trialr3, which will contain system-missing values where there were user-defined missing values in the original variables.
However, underlying mechanisms creating outlier data points are usually unknown. People might always find published or unpublished arguments to exclude or keep ‘unusual’ data points in analyses. Perhaps selection of data points will depend on baseline knowledge of data analyzers and, why not, personality profiles, like more or less critical people independent from education background. The impact of outliers will depend on the proportion of outliers in a data set (thus sample size dependent) and the values of the outliers in relation to the values frequently observed (median).
The approach can be used for multivariate data by calculating the limits on each variable in the dataset in turn, and taking outliers as observations that fall outside of the rectangle or hyper-rectangle. Three standard deviations from the mean is a common cut-off in practice for identifying outliers in a Gaussian or Gaussian-like distribution. For smaller samples of data, perhaps a value of 2 standard deviations (95%) can be used, and for larger samples, perhaps a value of 4 standard deviations (99.9%) can be used. To answer your question, how much outliers affect statistical analysis depends upon the analysis. Some methods are quite robust to outliers and some are quite sensitive.
If any of the component variables were missing, the value for avg or avgr was set to missing. For each pairof variables, corr used the number of pairs that had valid data. For the pair formed by trial1 and trial2, there were three pairs with valid data. For the pairing of trial1 and trial3 there were four valid pairs, and likewise there were four valid pairs for trial2 and trial3. Since this used all of the valid pairsof data, this is often called pairwise deletion of missing data.
Alternatively, you may realize that you have accidentally entered the same case in your dataset more than once and need to remove the extra case. You will notice that each of the columns is labeled “var.” The column names will represent the variables that you enter in your dataset.